Large language models (LLMs), a type of Artificial Intelligence, are the new trend, and ChatGPT is the most exciting addition. There is quite a stir in the industry that the Chatgpt API tends to have quick solutions to everything. And can replace humans involved with monotonous tasks shortly.
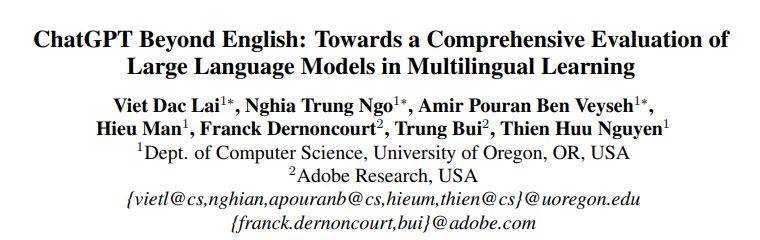
Especially educational institutes are banning the same, stating how students are using this particular to complete their homework. However, a research team from the Dept. of Computer Science, University of Oregon, had other questions in mind. As a resolution, they compared the functional abilities of ChatGPT with that of other AIs.
The main focus is “whether ChatGPT can solve (natural language processing) NLP problems in different languages with the same intent as English or whether it needs the development of more language-centric technologies?”
So to find adequate answers, the team went forward, testing ChatGPT as per 7 differential tasks
- Part-of-Speech (POS) Tagging
- Named Entity Recognition (NER)
- Relation Classification
- Natural Language Inference (NLI)
- Question Answering (QA)
- Common Sense Reasoning (CSR)
- Summarization
Covering 37 language types available with high, medium, low, and meager resources. Also, the team focused on ChatGPT’s zero-shot learning setting, which can improve reproducibility and interactions. What were the final results? Carry on with your reading task for a comprehensive answer.
Methodology: ChatGPT vs Other AIs (Large Language Models)
The goal is to evaluate the performance of ChatGPT and other Large Language Models while solving NLP (natural language processing) problems in various languages. The research team added seven different NLP tasks to add extra intensity to the study.
Also, the team did incorporate data for each language in the CommonCrawl corpus. The primary data was used to pre-train GPT-3 and classify the resource levels. In particular, a language is considered as
- High – greater than 1% (> 1%)
- Medium – between 0.1% and 1% (> 0.1%)
- Low – between 0.01% and 0.1% (> 0.01%)
- Extremely low resource – smaller than 0.01% (< 0.01%)
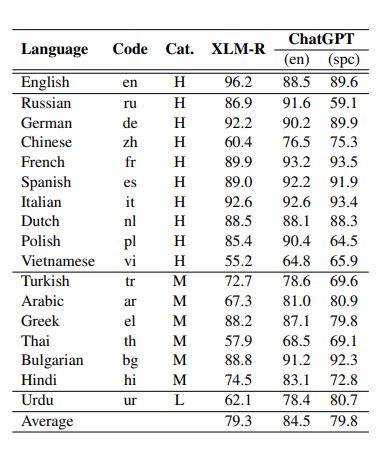
Further, the researchers went forward with a zero-shot learning setup for ChatGPT. To keep it different from the research so far, the team used single-stage prompting in this work, while it was two-stage prompting till now.
Moreover, for tasks that are in a non-English target language, the team went on to add task descriptions both in non-English and English language. This way, they were able to shed some light on how ChatGPT performs in multilingual settings.
The results of this work were obtained between the 1st of March and to 5th of April. Just after Open AI made ChatGPT available in its API. The company (OpenAI) enabled large-scale public requests seeking comprehensive evaluations.
To work on reproducibility, the team always cleared the query-specific conversations in ChatGPT.
The Findings: ChatGPT vs Other AIs (Large Language Models)
Here is what the research team found:
- ChatGPT, when compared with other large language models, the latter performed better. For the team, it looked more reasonable to develop smaller LLM models specific to particular tasks when solving NLP (natural language processing) problems in different language types.
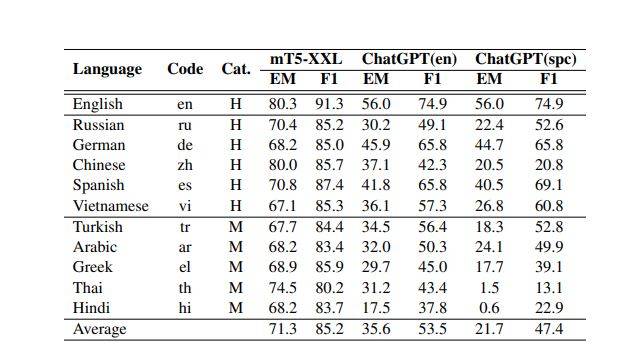
- Next, the ChatGPT model did better over different languages for the POS tagging part. The researchers also concluded that the AI model possesses high grammatical skills but is low on semantic reasoning when generating fluent texts for different languages.
- However, there are several other occasions where ChatGPT performs better with low-resource languages. The team suggests that data size and resources available are not the only factors dictating an LLM’s or ChatGPT’s task-specific performance. The given target or similarity to the language of the training data is an essential factor.
- Because the training data was predominantly in English for ChatGPT, the particular could better analyze and understand task-specific prompts in English.
Limitations To The Study: As Highlighted By The Team
The team itself highlights the following limitations:
- The researchers included only 37 languages. However, there is more that went on unexplored in the current work.
- Specific tasks in the research did not clever lower resource language.
- Tasks available with multilingual datasets have not been covered in this study.
- Including more functions in future studies will offer a comprehensive look at ChatGPT.
- The research is based on a zero-shot learning setting, which fails to showcase a comparison with other LLMs in the recent era.
- The recent work evaluates various models regarding their problem-solving over NLP, and there is adequate scope for betterment in this aspect.
Recently researchers from Microsoft have come up with their views on the successor of ChatGPT, GPT 4. You can get an overview of the same. “A Test Of The Ultimate Intelligence In GPT 4: Microsoft’s Overview”